COCO Traffic dataset
Overview
The Common Objects in Context (COCO) dataset is a popular dataset to train general object detectors and is often used as a benchmark to compare these detectors as well.
In this project the COCO dataset class traffic_light
is refined into three separate object classes: traffic_light_red
, traffic_light_green
, and traffic_light_na
. These new classes allow for a model to determine the state of a traffic light to determine whether a car can safely pass through an intersection
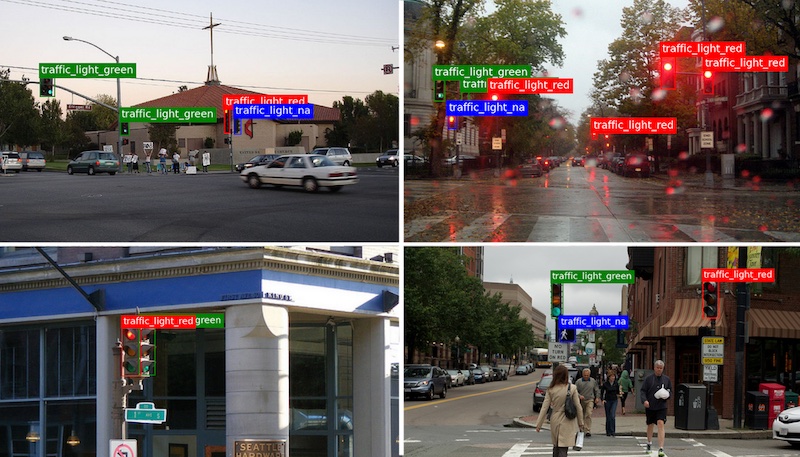
For more detailed information on the dataset and how it was curated, see the post.
Using the dataset
COCO Traffic is available on the official COCO dataset website and here. Follow the instructions below.
Downloads
A total of three subsets are available. These subsets are explained in more detail below.
Filename | Description | Size |
---|---|---|
train2017.zip | COCO training images | 18GB |
val2017zip | COCO validation images | 1GB |
LISA Traffic Light Dataset | Optional images for COCO Traffic Extended from the dataset LISA Traffic Light Images (Kaggle account required) | 5GB |
01_coco_refined.zip | Train and val annotations for COCO Refined | 158MB |
02_coco_traffic.zip | Train and val annotations for COCO Traffic | 64MB |
03_coco_traffic_extended.zip | Train and val annotations for COCO Traffic Extended | 65MB |
Setup
The setup varies for each of the three datasets since they require different files. For each follow the steps described below.
In all cases, create folders annotations
and images
at the root of this repository first. Then, download the images train2017.zip and val2017zip from the COCO website and extract them in the images
folder. Then your repository should look like this:
cocoTraffic/
├── annotations/
├── api/
│ ├── make_datasets.py
│ ├── make_yolo_labels.py
│ ├── ...
├── images/
│ ├── train2017/
│ ├── 000000000009.jpg
│ ├── ...
├── plots/
│ ├── ...
├── tools/
│ ├── ...
├── LICENSE
├── README.md
With this base setup choose the dataset that you need and follow the instructions.
1. COCO Refined
Full COCO 2017 dataset, with all traffic lights relabelled in training and validation dataset. Get the annotation files with the refined labels here and place them into the annotations
folder.
2. COCO Traffic
Subset of the train2017
images with classes which are related to traffic, and all traffic light images from val2017
. These images have been split into a training and validation set (split 80/20). The chosen classes are:
['traffic light', 'car', 'truck','bus', 'motorcycle', 'bicycle', 'person', 'dog', 'cat', 'stop sign', 'fire hydrant', 'train', 'traffic_light_red', 'traffic_light_green', 'traffic_light_na']
Ideal to train a smaller model to detect vehicles, pedestrians etc. Annotation files are available here.
3. COCO Traffic Extended
Extended COCO Traffic with images of traffic lights from the LISA Traffic Light dataset. We labelled these images to include all classes from COCO Traffic
. Annotation file
Tools
To label the data, we created and/or used the following tools.
make_yolo_labels.py
- Creates labels for yolov5 from COCO annotation files.
dataLabeller
- Tool which iterates through COCO annotations and lets you change their category id. Used to relabel the traffic lights.
makesense
- Makesense is a freely available annotation tool which we used to label the images in the LISA Traffic Lights dataset. We include a file which converts the output from makesense.ai
into a COCO dataset annotation file.
prelabeller
- DETR model to label data with COCO classes. We used it to pre label the LISA Traffic Light images.
Project links
References
- Tsung-Yi Lin and Michael Maire and Serge Belongie and Lubomir Bourdev and Ross Girshick and James Hays and Pietro Perona and Deva Ramanan and C. Lawrence Zitnick and Piotr Dollár. Microsoft COCO: Common Objects in Context. 2015. https://arxiv.org/abs/1405.0312
0 Comments